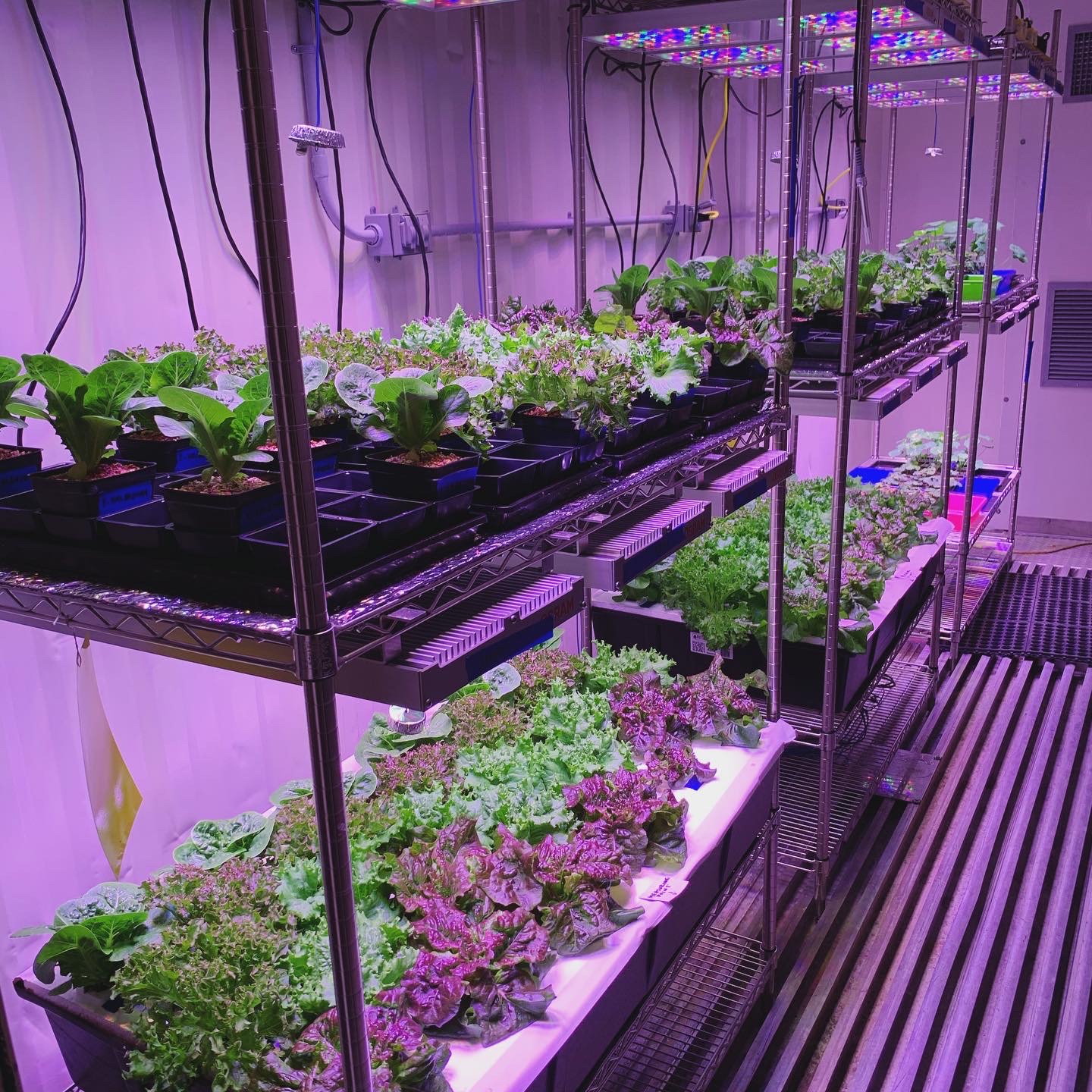
Controlled Environment Agriculture Engineering
AI and automation research for agricultural production in controlled environments
Tailored technologies for controlled environment agriculture
The Controlled Environment Agriculture (CEA) Engineering Lab at Dallas aims to pioneer research in automation and artificial intelligence (AI) technologies tailored for CEA.
We are committed to developing innovative and intelligent solutions that minimize labor requirements, provide decision support to optimize resource usage, and reduce production costs to enhance the sustainability of the CEA industry.
Research areas of focus
Areas of research focus in controlled environment engineering include:
- AI-assisted computer vision systems to monitor various aspects of crop health and growth, such as biotic and abiotic stresses, organ development and growth, and yield prediction.
- IoT-edge AI systems for real-time monitoring and precision management of different production components such as nutrients, pests/diseases, and irrigation.
- Robotics and intelligent automation solutions to reduce labor requirements for various production operations, including transplanting, harvesting, and sorting.
- AI-assisted microclimate controllers to optimize recipes (light, temperature, CO2, humidity) for creating the ideal environments for cultivation.
Digital Twins for Data-Driven Greenhouse Management and Controls
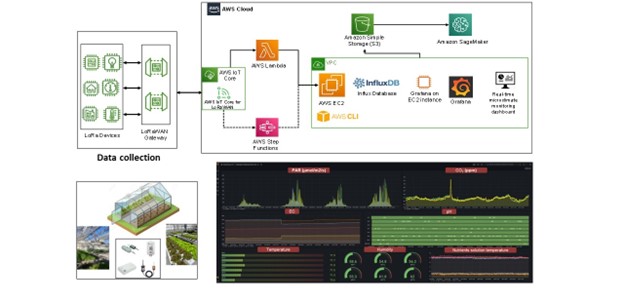
Optimizing resource utilization (water, energy, and nutrients) in CEA offers several advantages, yet achieving optimized resource allocation strategies (when, where, and how much) remains a challenge. Digital Twins (DT) could be used to examine historical data on crop growth, climate conditions, and resource usage to predict future growth patterns and optimize resource allocation. For example, it can help determine when to increase light intensity, adjust heating or cooling systems, add CO2, and harvest crops for maximum yield. Our research program developed a DT framework for precision resource management within CEA, serving as a fundamental tool for real-time monitoring, management, and controls to optimize crop productivity while reducing input costs. Our DT framework aggregates data pipelines, including microclimate and crop growth, for predictive microclimate and growth monitoring and decision support. By synchronizing actual and simulated conditions, it enables real-time analytics of microclimate and crop growth in a closed-loop monitoring and management system.
AI-assisted Decision System for Planting Density Optimization in Greenhouse Hydroponics
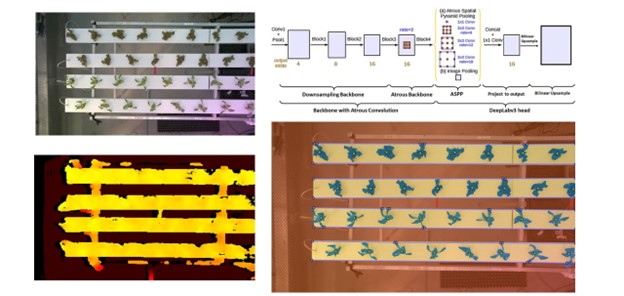
Plant spacing in greenhouse hydroponics is a critical design parameter influenced by crop type and production technology. Proper spacing must be carefully monitored to ensure high planting density, high crop yield, and quality, as improper spacing can lead to foliage occlusion or overlapping, reducing photosynthesis. Conversely, too much space between plants can result in lower space use and light use efficiency, resulting in inefficient resource utilization. Our research program is dedicated to developing computer-vision algorithms for plant monitoring to optimize plant spacing and improve plant density in greenhouse hydroponics. By achieving a balance between plant overlap and gaps, we aim to maximize greenhouse space utilization, significantly enhancing overall productivity and crop quality in CEA.
High-Throughput Phenotyping of Lettuce for Precision Resource Allocation
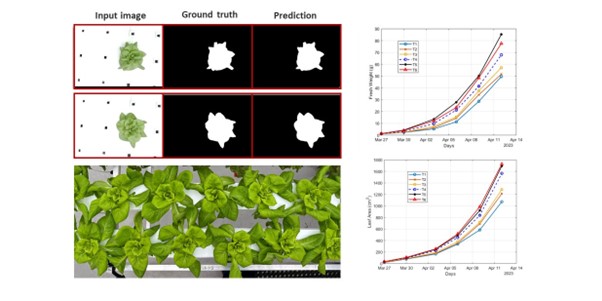
Monitoring crop growth is crucial for optimizing management strategies and maximizing greenhouse production. Traditionally, crop monitoring has been done manually, making it impractical to collect daily data necessary for actionable insights to achieve high yields. To address this, we developed an innovative, non-destructive, end-to-end approach using deep learning to predict lettuce growth parameters such as leaf area, fresh weight, dry weight, plant diameter, and plant height. Our model achieved high accuracy with R² values of 0.968 for fresh weight, 0.953 for leaf area, 0.943 for dry weight, 0.906 for plant diameter, and 0.965 for plant height. To underscore the model’s robustness, we validate it under various conditions, encompassing variations in nutrient concentration and temperature conditions. These validations substantiate the prediction model’s efficacy and hold promise for data aggregation and predictive analytics to assist growers in decision-making for optimum resource optimization.
Deep Learning for Strawberry Maturity Classification and Yield Estimation
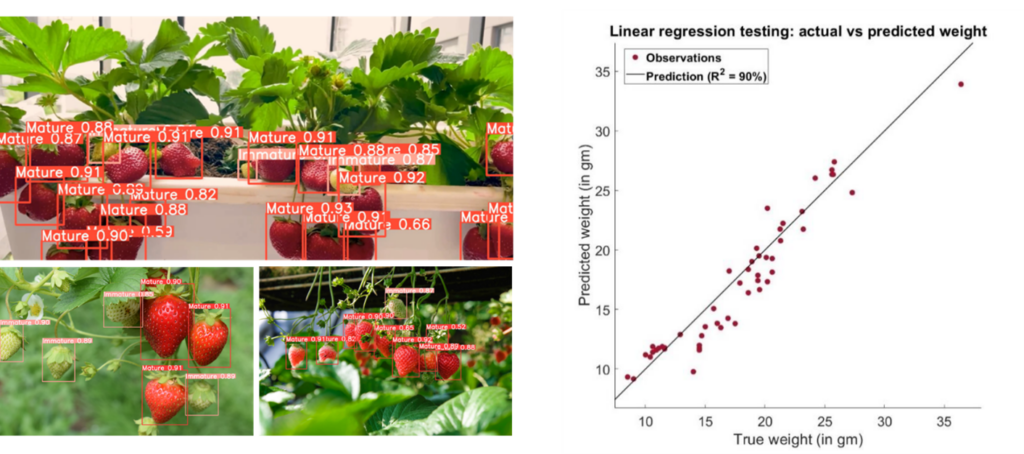
Harvesting strawberries is a labor-intensive operation, constituting about 40% of total production costs. CEA is an intensive production system; the labor requirement is significantly higher than in field agriculture. Rising labor costs and decreasing availability pose sustainability challenges. However, automation and robotics offer potential solutions by reducing labor dependency. Essential tasks like sensing the maturity of the fruit, estimating size and weight, and counting strawberries based on maturity levels are critical for robotic operations in CEA. Our team developed AI models for automated harvesting and counting, achieving a mean average precision of 92.1% for fruit maturity classification and an R² of 0.90 for yield prediction across different maturity levels. The results demonstrate the proposed models can work effectively without compromising the precision and satisfying real-time requirements.
Cyber-Physical System for Greenhouse Plant Disease Detection
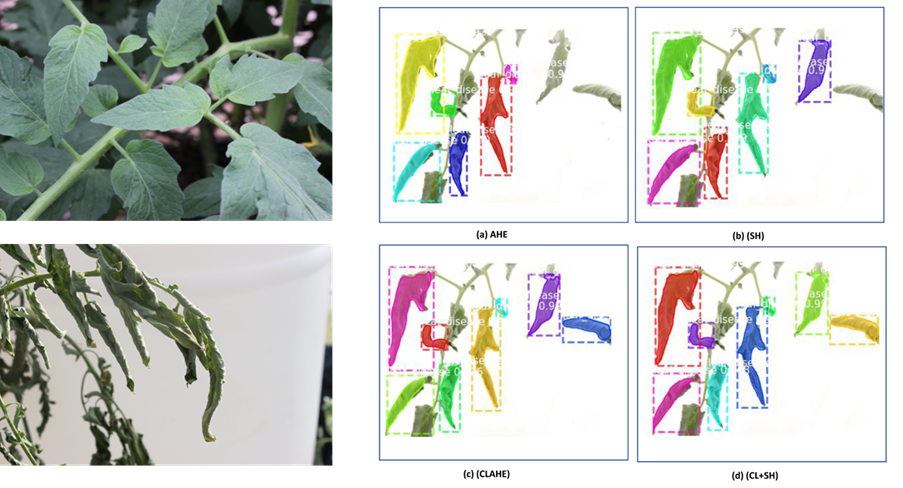
Crop scouting for disease detection and diagnosis is the first critical step for the successful control of plant diseases. However, manual crop scouting is a laborious and time-consuming operation. Early identification of vegetable disease using automated computational systems is essential for improving detection accuracy in a timely manner. Our team developed an innovative framework in which the integration of image analysis, data imbalance handling, and AI-assisted models was evaluated for the early detection of diseases in tomato plants in a greenhouse environment. The evaluations indicated that the Generative Adversarial Networks (GANs)-based approach for resampling performed best, with an average classification accuracy of 97.69% for the target disease. The developed algorithm was deployed on edge devices (Jetson Nano, Raspberry Pi) for autonomous disease monitoring and management in the tomato greenhouse. The work will enable the development of future precision autonomous systems to apply spraying chemicals in the correct amount and in the right place, which will reduce environmental pollution and serve public interests.
Robotic Harvesting System for Greenhouse Hydroponic Lettuce
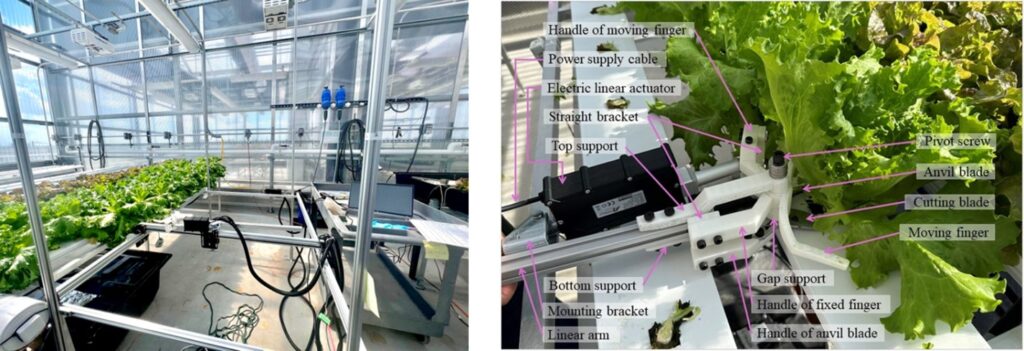
Lettuce is most widely consumed as fresh-market leafy green vegetables in the U.S. Greenhouse lettuce, meanwhile, is at a much earlier stage of its evolution, with a cost of production still significantly higher than that of field-grown lettuce. Labor accounts for more than one-third of the total production cost. Decreasing labor availability and increasing labor costs are the two most critical challenges in greenhouse lettuce production. Thus, automation to reduce labor requirements is required for the industry’s sustainability. We developed a prototype of a robotic end-effector for harvesting greenhouse hydroponic lettuce. The harvesting end-effector was developed using an actuator, a pair of blades, and a pair of fingers, which was then integrated into an automated harvesting system incorporating a control system and a three-directional linear manipulator system. Validation experiments were conducted for different lettuce cultivars, achieving the success rates for cutting and holding as 92% and 90%, respectively. Our harvesting end-effector prototype serves as a pivotal component in lettuce harvesting automation.
Program Faculty and Staff
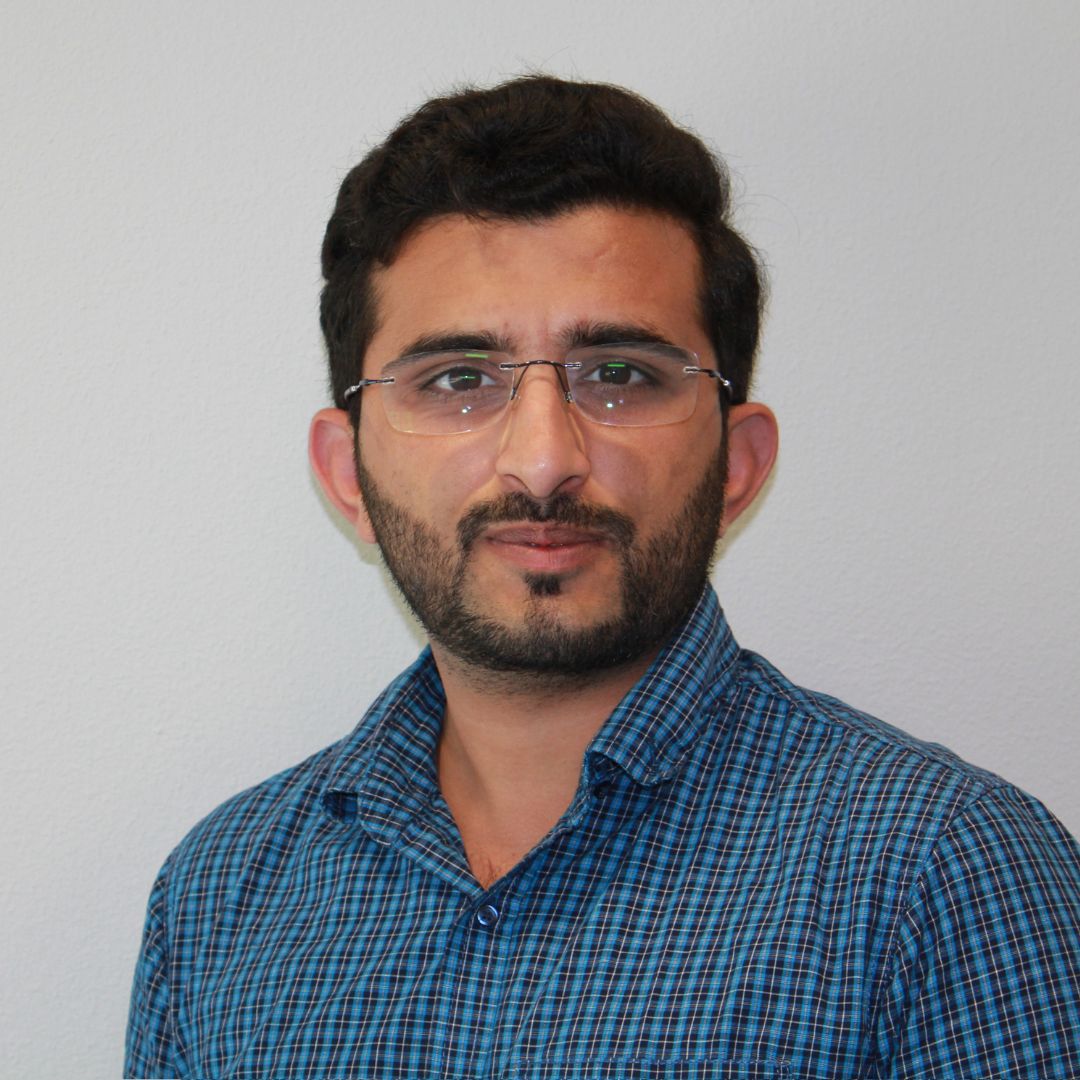
Dr. Azlan Zahid, Ph.D.
Assistant Professor, Principal Investigator
Publications
A full selection of Dr. Zahid’s publications is available at TAMU Scholars along with information about researchers and peer-reviewed publications across The Texas A&M University System.
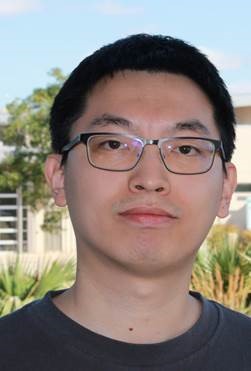
Yibin Wang
Postdoctoral Research Associate
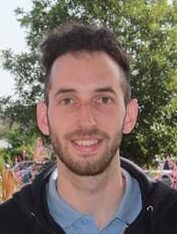
Cristian Bua
Visiting Scholar (from Italy)
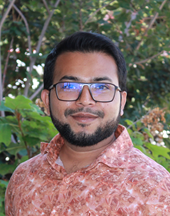
Al Bashir
Graduate Assistant
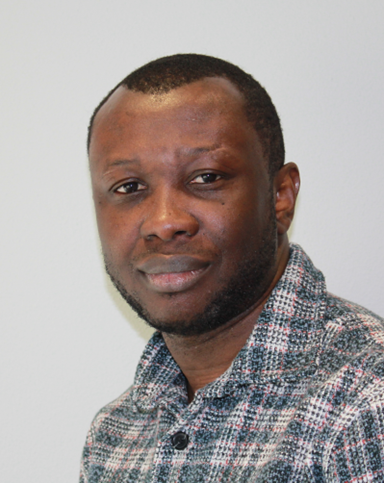
Mike Ojo
Research Associate
Explore Controlled ENvironment Horticulture at Dallas
The AgriLife Extension Urban Horticulture program is part of a comprehensive effort at the Texas A&M AgriLife Research and Extension Center at Dallas to advance all aspects of crop production in controlled environments — delivering nutritious, delicious, and resilient food crops that are economically and environmentally sustainable.